ARTICLES
DeepETA: How Uber Predicts Arrival Times Using Deep Learning | 15 min read | Machine Learning & AI | Uber Engineering Blog
For several years, Uber used gradient-boosted decision tree ensembles to refine ETA (arrival time predictions). Eventually, Uber’s Apache Spark™ team reached a point where increasing the dataset and model size using XGBoost became untenable. To continue scaling the model and improving accuracy, they decided to explore deep learning. To justify switching to deep learning, they needed to overcome three main challenges:
- Latency: The model must return an ETA within a few milliseconds at most.
- Accuracy: The mean absolute error (MAE) must improve significantly over the incumbent XGBoost model.
- Generality: The model must provide ETA predictions globally across all of Uber’s lines of business such as mobility and delivery.
To meet these challenges, Uber AI partnered with Uber’s Maps team on a project called DeepETA, which is presented in this article.
Accelerate testing in Apache Airflow through DAG versioning | 7 min read | Apache Airflow, Big Data, Data Science | Hilmi Yildirim & Stefan Haase | Zalando Engineering Blog
How did Zalando enable the versioning of the performance marketing pipeline which is based on Apache Airflow?
- The Versioning is necessary to enable more convenient simulation and testing.
- They modified the Airflow DAGs class and used the Packaging DAGs feature of Apache Airflow to make it possible to have multiple versions of the same DAGs on a single server.
- The deployment takes less than 1 minute compared to up to 30 minutes when you create a new Airflow server for the deployment.
- Every Pipeline version can use a dedicated Data Environment.
Adidas Data Mesh Journey: Sharing data efficiently at scale | 11 min read | Data Platforms | Jose Luis Alcala | Adidas Engineering Blog
Insights of applying Data Mesh principles in a prototype to share data at scale.
Evolution of ML Fact Store | 11 min read | ML | Vivek Kaushal | Netflix Technology Blog
This post focuses on the large volume of high-quality data stored in Axion — fact store that is leveraged to compute ML features offline. Netflix built Axion primarily to remove any training-serving skew and make offline experimentation faster. This post will show how its design has evolved over the years and the conclusions after building it.
TUTORIALS
Airbyte is in the air - data ingestion with Airbyte | 11 min read | Airbyte | 👏 Bartosz Konieczny | GetInData Blog
“In our analysis we decided to focus on the offline data ingestion with Airbyte. Why this one in particular? Mostly for its business model. Airbyte is an Open Source project backed by a company. It has a lot of available connectors, supports different data ingestion modes and integrates pretty well with Apache Airflow and dbt.”
NEWS
Introducing Unistore, Snowflake’s new Workload for Transactional and Analytical Data | 7 min read | Snowflake | Carl Perry | Snowflake Blog
Snowflake introduces Unistore, which will allow organizations can use a single, unified data set to develop and deploy applications, and analyze both transactional and analytical data together in near-real time.
Did you watch the Snowflake summit? Unistore looks very promissing!
DATA ODDITY
Is LaMDA Sentient? — an Interview | 20 min read | AI | Blake Lemoine
Blake Lemoine - a Google engineer who was suspended for alleging that LaMDA (Language Model for Dialogue Applications) is sentient, posts conversations between him and Google’s system for building chatbots. You can read it here.
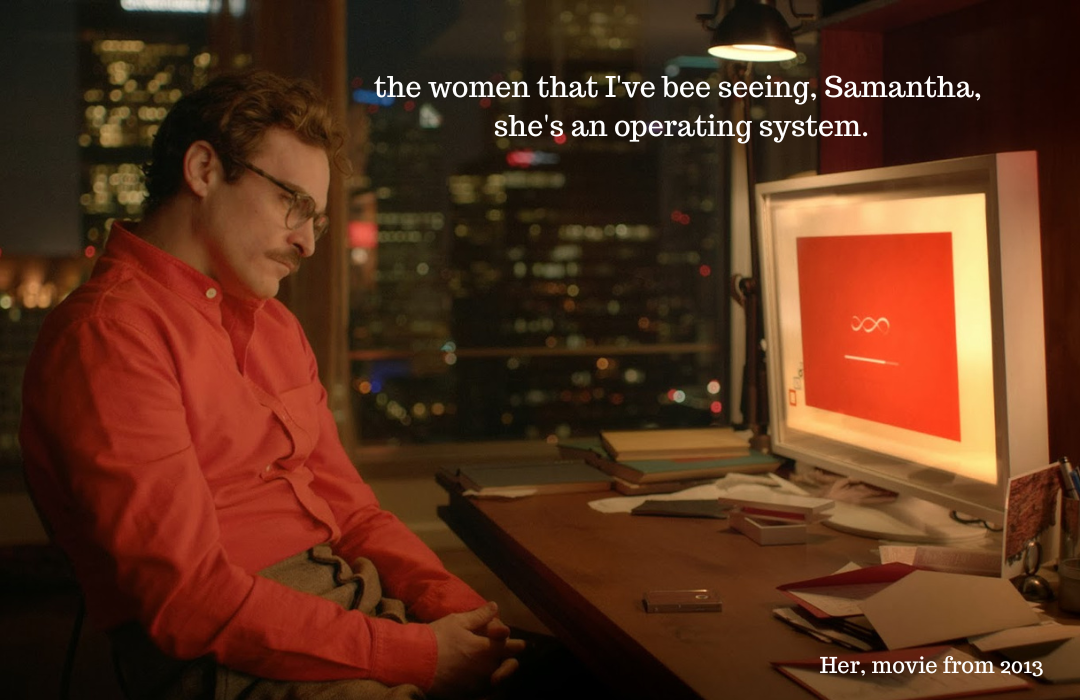
DATAtube
MLOps Critiques. Matthijs Brouns. MLOps Coffee Sessions| 49 min | MLOps.community
“MLOps is too tool-driven, don't let FOMO drive you to pick the latest feature/model/evaluation/ store, but pay closer attention to what you actually need to release more safely and reliably.”
MLOps implemented - How we combine the cloud and open-source to boost data scientists work.| 35 min | GetInData
How projects of ML models training can go from zero to production in much shorter time?
How to achieve superior performance, high code quality, training repeatability and governance?
How platform mixes best-of-breed cloud managed services with a small number of powerful open-source components (e.g. Kedro, MLflow, Seldon) to get extra functionality that data scientists and their ML models need?
All on case studies.
PODCAST
Cloud Native Storage, with Alex Chircop | 43 min | Kubernetes Podcast
Talk with: Alex Chircop, co-chair of the CNCF Storage Technical Advisory Group (TAG), as well as founder and CEO of Ondat (formerly StorageOS) on why no app is truly stateless, and how data is the new storage.
Building a Holistic Data Science Function at New York Life Insurance | 37 min | DataFramed
Interview with Glenn Hofmann, Chief Analytics Officer at New York Life Insurance.
How did he build NeW York Life Insurance’s 50-person data science and AI function?
How do they utilize skillsets to offer different career paths for data scientists, building relationships across the organization?
Find out in the podcast.
CONFS AND MEETUPS
Google Warsaw Cloud Meetup | 28 June | Online
Eventarc: asynchronous events in Google Cloud
“Eventarc is a product that helps to build event-driven architecture without having to implement, customize or maintain the underlying infrastructure. In this talk you will learn about Eventarc, how it can be used, and how the Warsaw engineering team builds UIs like this, going from the idea right trough to the launch.”
Speakers: Maciej Szarliński and Sasha Sabov
DataMass Gdańsk | 30 September | Call For Presentation till 30 June
Big Data, Data Science, Machine Learning and AI biggest conference in the Northern Poland.